ESA and TU Delft Collaborate on Autonomous Spacecraft Control
The European Space Agency’s (ESA) Advanced Concepts Team and the Micro Air Vehicle Laboratory (MAVLab) at Delft University of Technology (TU Delft) have joined forces to test neural-network-based AI control systems for next-generation space missions. The research, published in Science Robotics, involves racing drones against the clock at TU Delft’s ‘Cyber Zoo’ to evaluate the performance of these AI systems.
Optimizing Spacecraft Operations with Neural Networks
Dario Izzo, scientific coordinator of ESA’s ACT, explains that the collaboration aims to utilize trainable neural networks for the autonomous oversight of various demanding spacecraft maneuvers, such as interplanetary transfers, surface landings, and dockings.
“In space, every onboard resource must be utilized as efficiently as possible – including propellant, available energy, computing resources, and often time,” Izzo notes. “Such a neural network approach could enable optimal onboard operations, boosting mission autonomy and robustness.”
Drone Racing as a Testing Ground
To test the neural network approach in the real world before planning actual space missions, the researchers settled on drone racing as the ideal gym environment. Drones competed to achieve the best time through a set course within the Cyber Zoo at TU Delft, a 10×10 m test area maintained by the university’s Faculty of Aerospace Engineering.
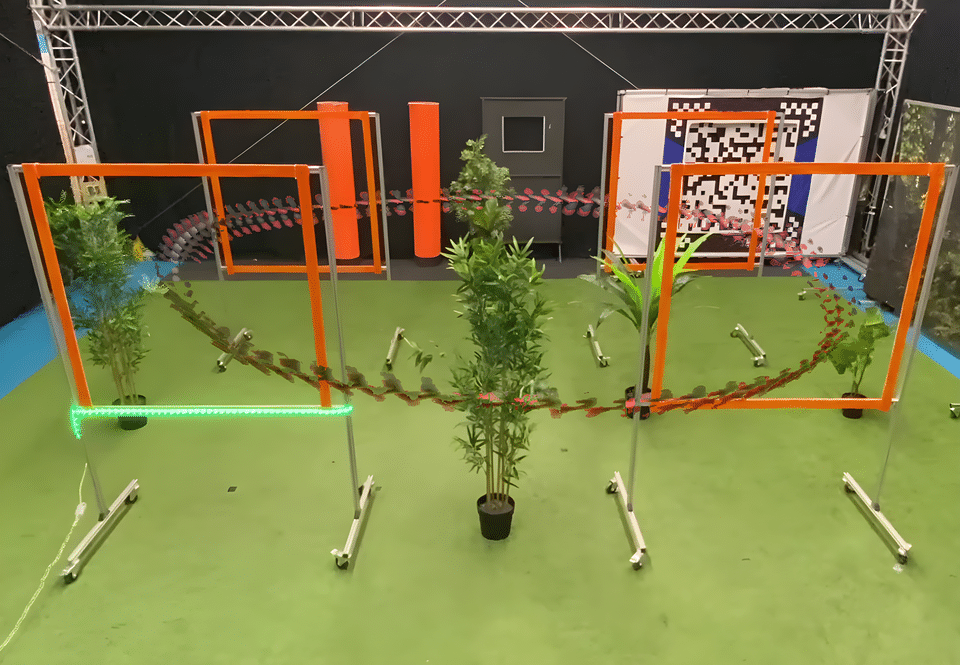
Comparing Traditional and Neural Network Approaches
Sebastien Origer, an ACT Young Graduate Trainee, explains that traditional spacecraft maneuvers are planned in detail on the ground and then uploaded to the spacecraft for execution. However, the space environment is inherently unpredictable, with potential for unforeseen factors and noise. The alternative end-to-end Guidance & Control Networks (G&C Nets) approach involves all the work taking place on the spacecraft, continuously replanning its optimal trajectory based on its current position, proving to be much more efficient.
Synergies Between Drones and Spacecraft
Dario Izzo comments on the synergies between drones and spacecraft, despite the faster and noisier dynamics involved in flying drones.
“When it comes to racing, obviously the main scarce resource is time, but we can use that as a substitute for other variables that a space mission might have to prioritize, such as propellant mass,” Izzo explains.
Overcoming the Reality Gap
Christophe De Wagter, principal investigator at TU Delft, highlights the main challenge in bringing G&CNets to drones: the reality gap between actuators in simulation and reality.
“We deal with this by identifying the reality gap while flying and teaching the neural network to deal with it,” De Wagter says. “For example, if the propellers give less thrust than expected, the drone can notice this via its accelerometers. The neural network will then regenerate the commands to follow the new optimal path.”
Building Trust and Planning Future Space Missions
Sebastien Origer emphasizes that the use of drones in this research represents a way to build TRUST, develop a solid theoretical framework, and establish safety bounds ahead of planning an actual space mission demonstrator.
“There’s a whole academic community of drone racing, and it all comes down to winning races,” Origer says. “For our G&CNets approach, the use of drones represents a way to build trust, develop a solid theoretical framework and establish safety bounds, ahead of planning an actual space mission demonstrator.”
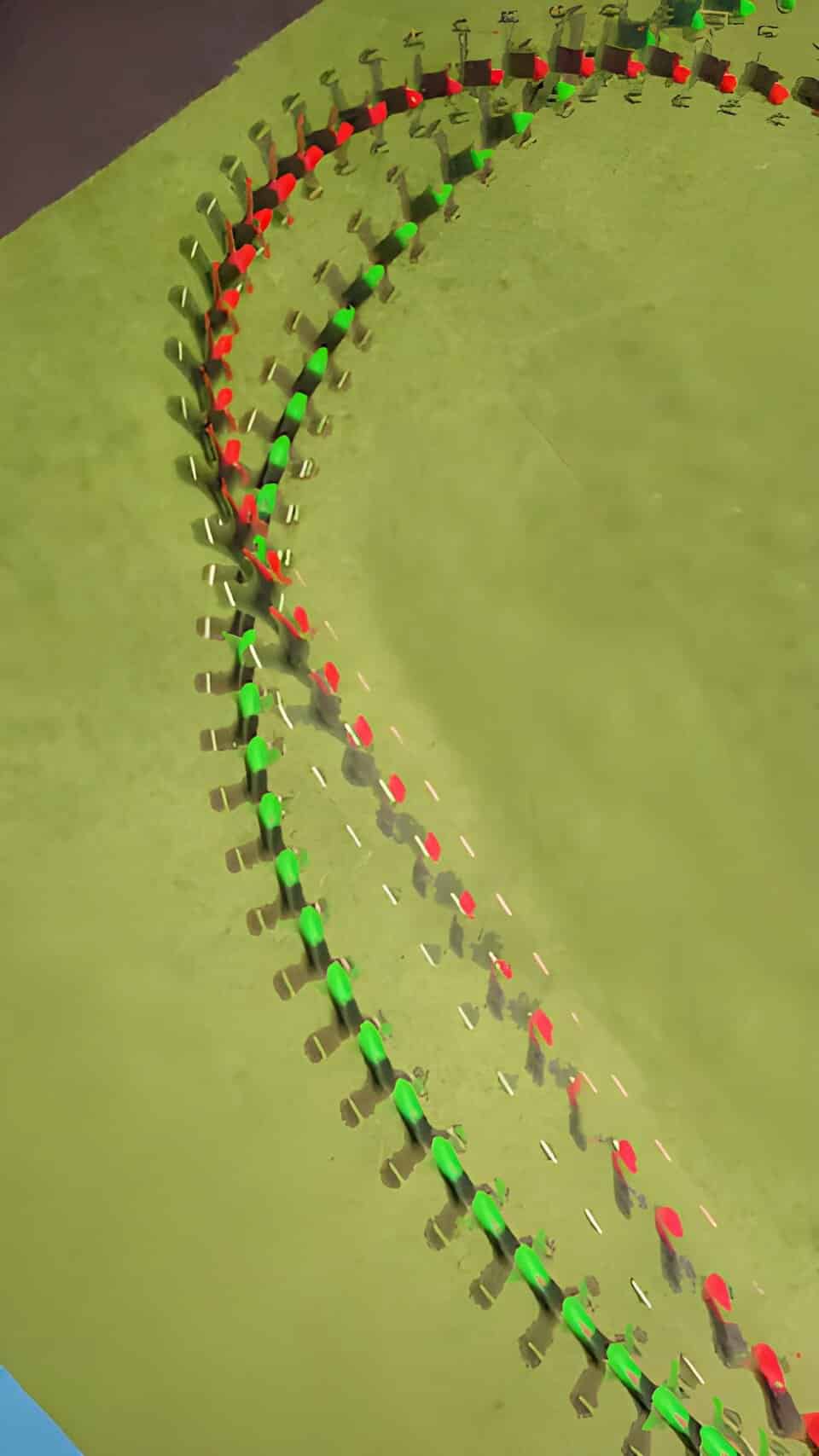
DroneXL’s Take
The collaboration between ESA and TU Delft showcases the potential of neural-network-based AI control systems in optimizing spacecraft operations and enhancing mission autonomy. By leveraging the fast-paced and dynamic environment of drone racing, researchers can test and refine these AI systems in real-world conditions, paving the way for their future application in space missions.
As the Drone Industry continues to evolve, the insights gained from this research could also have implications for the development of more efficient and autonomous drones for various applications on Earth. The ability to continuously replan optimal trajectories based on real-time data could lead to significant improvements in drone performance and adaptability in challenging environments.
Discover more from DroneXL
Subscribe to get the latest posts to your email.
+ There are no comments
Add yours